Since the discovery of neurons, neuroscientists have kept inventing new ways to visualize patterns of brain activity. With fMRI technology, they have found a tool worthy of their ambition to literally decode brains. Neuroscientists can now predict and accurately connect neural functions with cognitive behaviors by running computing models like a data scientist. But according to Russell. A. Poldrack in The New Mind Readers, these techniques have the same biases and limitations as artificial intelligence models, and require a rigorous scientific methodology.
Here is the history and implications of this technology, and what it can teach us about the brain-computer interface.
The history of brain scan technologies
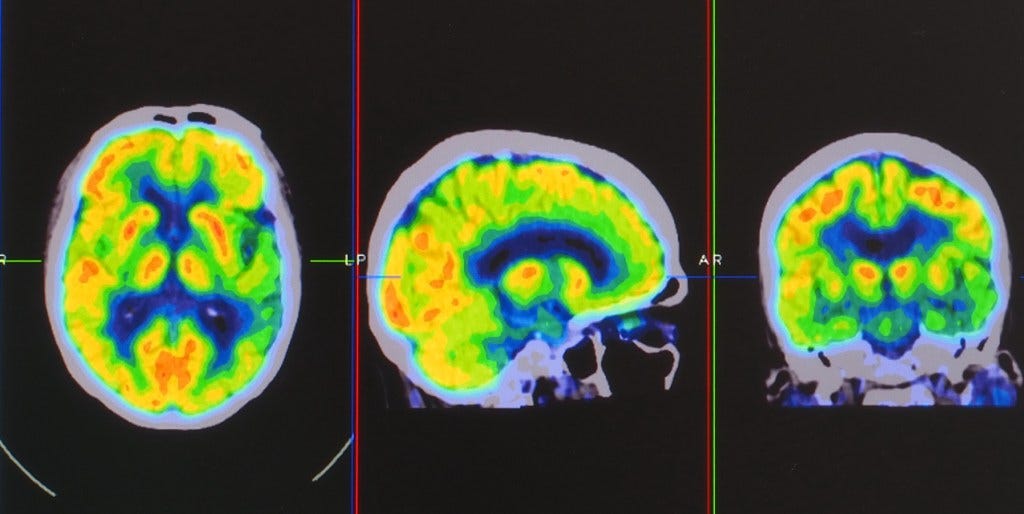
While neuroscientists noticed in the early 20th century that the brain blood-flow varied significantly, they did not yet have a way to measure these changes. They found one with the invention of position emission tomography in the 1980s.
With such technology, researchers were now able to visualize changes in neuronal activity through radioactive tracing and detection of photon emission. Since these photons are most degraded where neurons consume the most glucose, they are indicative of their activity.
However, the first studies using this method faced a big problem: each individual has a brain of different sizes and structures, which introduce considerable variations. Moreover, PET scans have a very low spatial and temporal image resolution. They detect areas at least one millimeter wide and take 10 seconds to collect enough data to form an image. So the technology had a range still quite limited.
The concept of magnetic resonance imaging (MRI) helped create a more accurate picture of the brain based on the vibration of atomic nuclei. As an MRI scanner sends signals to many locations at varying speeds, it can form an image by decoding different frequency bands. However, the researchers used a contrast agent that could be dangerous for the health of the subjects. Fortunately, by noticing that MRI signals are sensitive to the level of oxygenation of the blood circulating in the brain, that many teams of researchers came up with the idea of MRI that detects the activity of the brain (fMRI) in the 90s.
This technology would then provide a real way to decode the signals of neuron activities in space and time.
Brain activity and data science
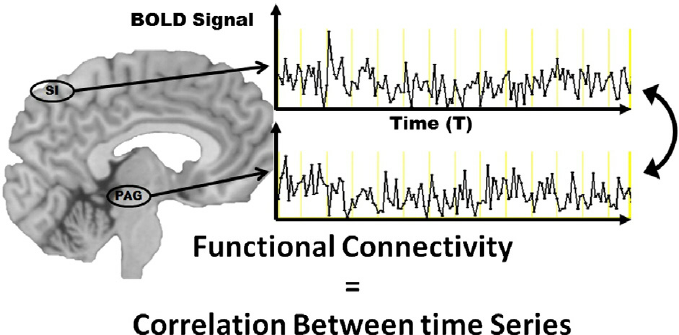
While neuroscientist Jim Haxby was studying parts of the brain sensitive to the perception of faces, he also noticed how sensitive these sections were to other objects. These neural networks, so specific to faces, were actually not only specific to faces.
This gave Haxby the idea to focus not only on the regions of fMRI active under a certain condition but on the overall signals of each region.
The traditional method of neuroscientists had been to infer statistically selective regions by spotting the most active signal regions. Now, the goal was to infer the purpose of the common activity pattern of selective regions.
By assessing the regular behavior of each brain section for various objects, Haxby then tried to guess the perceived object by how they react to it. He had subjects perform perception tests on objects such as a chair or a bottle to retain the usual neuronal perception structure of the neurons. Then, he tried to infer the image perceived by comparing the structure of perception with the one already performed. This meticulous work allowed him to decode with 90% accuracy any object perceived by the patients.
He concluded that the neural networks don’t react specifically to one object, but were statistically distributed to respond with different proportions to many objects. A kind of statistically relevant correlation.
Bharat Biswal came to the same conclusion, when he noticed how the motor cortex of patients lying motionless during an fMRI still had correlated signals from each other. These areas were linked by “functional connectivity”, reacting and firing together. The brain is crisscrossed with “connectdomes”, structural connections between different parts, which define its uniqueness. These neural networks form a “small-world” where a few elements are extremely linked by very scattered connections forming a hub. This work led to techniques that allow to some extent the prediction of brain movements through fMRI.
Visualizing and reading brain signals
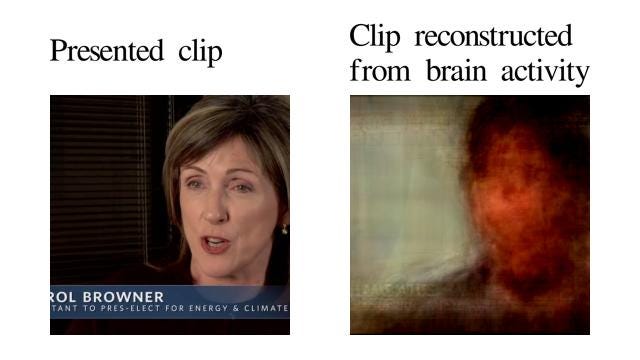
The decoding attempts presented so far consisted in guessing the perceived object by comparing it with experiments already done on the object, and this for the same person.
Teams of neuroscientists at Rotgers University have tried to take this principle a step further, by training a computational model to predict any object perceived by any person from much larger data sets. This method based on machine and statistical learning aimed at predicting people’s thinking based on a cross-validation of neural models.
They collected data from 130 patients performing many tasks and ran them through a learning algorithm. They removed the data of one person and tried to predict it from the data of the 129 other patients (a technique called “leave one out”). In this way, they were able to guess with 80% accuracy what the people were doing just from their neural activity pattern.
Thomas Naselaris and his colleagues at Berkeley University applied this technique to the recognition of images perceived by the subjects. They collected fMRI data from the front of many images. The only difference is that they then fed the model with millions of images from the internet as prior knowledge. Thus, the model was able to reconstruct the images that the subject was supposed to see by relating them to the most similar images in its database. It was thus literally able to read the visual thought of the person. And the results are impressive.
The limitations of brain decoding
But despite all these successes, these statistically based scientific inferences must be taken with epistemic caution. The fMRI analyses measure hundreds of thousands of small cubes called voxels. To find a meaningful response from a part of the brain that is not due to random variability, statistical tests must be performed that allow for specific margins of error. Thus, there are risks of true or false positives to be gauged, as when researchers find a significant response in one of their experiments while the signal is insignificant in the data in general when these experiments are replicated several times. Therefore, one must be able to repeat the experiment hundreds or even thousands of times to be sure of the results. But this precaution is not always taken.
Another problem in the use of fMRI statistics is the so-called “non-independence” statistical error. Researchers tend to select the data and results that best suit their study. For example, they may focus on the experiments where the voxels show the strongest correlations, among all the statistical tests. And this comparatively can make the result much better. But this is why it is necessary to agree in advance on the set and scope of the data studied, independent of the data we are going to computerize.
Despite these crucial issues, current neurosciences can now use computational technologies to make sense of the activity and structure of the brain. Because the human brain is defined by signals distributed geographically among many neural networks, we can compile neural signal in a statistical way and so make sense of their function. And there is nothing better for this than machine learning. Learning machines make us read brains that learn!